
Daniel Bojar Group
Short description
Associate Senior Lecturer. Research on glycan-focused machine learning, systems biology, and synthetic (glyco)biology.
Daniel Bojar chosen for the 30 under 30 Europe list 2022 by Forbes Magazine
Each year the Forbes Magazine selects a list of 30 talents under 30 in Europe within categories such as finance, art & culture and impact. Assistant Professor Daniel Bojar, project leader within WCMTM, has been chosen as this years winner within the category of Science & Healthcare. Read more about Daniel Bojar and the Forbes 30 under 30 Europe list here: 30 under 20 Europe 2022
Research summary
Our overall goal is to use computational and experimental resources to better understand the intricate roles of glycans in biology and integrate glycobiology into commonly used high-throughput systems biology methods. Glycans, or complex carbohydrates, are a fundamental biopolymer next to DNA, RNA, and proteins and adorn other biomolecules or occur by themselves. Among biological sequences, glycan exhibit the highest diversity and the distinction of being the only non-linear biological sequence that is, furthermore, outside the central dogma of molecular biology. Glycans exercise crucial - yet insufficiently understood - roles in development, immunity, pathogenesis, cancer, and many more areas. Combining the best of both worlds, glycans have the complexity of a biological language comprising monomeric building blocks and the dynamicity of a post-translational modification, making them largely responsible for phenotypic plasticity.
The two main difficulties facing glycobiology today are the inability of extracting generalizable, mechanistic, or actionable insights from these highly diverse glycan sequences as well as the shortage of known glycan sequences due to the experimental difficulties of working with glycans. We are working on overcoming these difficulties to reap the rich rewards promised by the omnipresence of glycans in biological mechanisms and nearly all diseases. For this, we have developed deep learning models for glycobiology that, together with other bioinformatics approaches, can extract functional insights from glycan sequences for a more holistic understanding of molecular biology. We are continuing the development and application of new and improved analysis methods for glycobiology at scale, both computationally as well as experimentally. Additionally, we are constructing a platform to transform glycobiology into a true high-throughput discipline by interweaving it with current systems biology methods, lifting the sequence bottleneck that is currently limiting the scope of glycobiology. Our expertise in mammalian synthetic biology and protein engineering then allows us to use the insights gained by our deep learning models to modify glycans in situ and capitalize on their important roles in new therapeutic modalities in biomedicine.
Research tools and resources
We apply a wide range of methods, both computationally as well as experimentally. Our computational repertoire extends to the analysis of systems biology data, bioinformatics techniques, machine learning / deep learning, and the emerging area of glycobioinformatics. Experimentally, we engage in synthetic biology / genetic engineering in mammalian cells and bacteria, including techniques such as CRISPR/Cas9 gene editing, as well as in systems biology methods such as RNA-seq or glycomics.
We are especially interested in developing and applying methods for understanding the overarching role of glycans in biology and integrating glycobiology into current high-throughput systems biology efforts. Particularly, we are at the forefront for constructing glycan-focused machine learning algorithms. The integration of a computational "dry" lab and an experimental "wet" lab enables us to test our predictions and rapidly investigate new mechanisms that broaden our understanding of glycans and have considerable biomedical implications.
Artificial Intelligence and Machine Learning
We routinely develop and use AI to facilitate our work on understanding complex carbohydrate function. One example would be our model LectinOracle, a deep learning model that uses large pre-trained protein language models and graph neural networks that we developed for glycans. We showed that LectinOracle can generalizably predict protein-carbohydrate interactions and can be used in high-throughput applications such as studying the microbiome or viral epidemics. We also developed machine learning models that could predict single-cell glycan expression from scRNA-seq data in certain scenarios and we are currently deeply engaged in developing and applying AI to the analysis of mass spectrometry data, for the automation of glycomics data analysis.
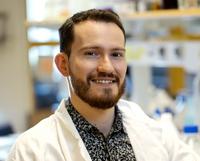
Contact Information
Selected Publications
06/2021 Thomès, L., Burkholz, R., and Bojar, D. Glycowork: A Python package for glycan data science and machine learning. Glycobiology, cwab067.
06/2021 Burkholz, R., Quackenbush, J., and Bojar, D.
Using Graph Convolutional Neural Networks to Learn a Representation for Glycans.
Cell Rep, 35:109251.
10/2020 Bojar, D., Powers, R.K., Camacho, D.M., and Collins J.J.
Deep-Learning Resources for Studying Glycan-Mediated Host-Microbe Interactions.
Cell Host Microbe, 29:132-144.
04/2020 Bojar, D., Powers, R.K., Camacho, D.M., and Collins J.J.
SweetOrigins: Extracting Evolutionary Information from Glycans.
bioRxiv, doi:10.1101/2020.04.08.031948.
01/2020 Bojar, D., Camacho, D.M., and Collins J.J.
Using Natural Language Processing to Learn the Grammar of Glycans.
bioRxiv, doi:10.1101/2020.01.10.902114v1.
04/2019 Kim, H.*, Bojar, D.*, and Fussenegger, M. A
CRISPR/Cas9-based central processing unit to program complex logic computation in human cells.
Proc Natl Acad Sci USA, 9:7214-7219. Co-first authorship.
06/2018 Bojar, D., Scheller, L., Charpin-El Hamri, G., Xie, M., and Fussenegger, M.
Caffeine-inducible gene switches controlling experimental diabetes.
Nat Commun, 9:2318.
04/2018 Kojima, R.*, Bojar, D.*, Rizzi, G., Charpin-El Hamri, G., El Baba, M., Saxena, P., Auslaender, S., Tan, K.R., and Fussenegger, M.
Designer exosomes produced by implanted cells intracerebrally deliver therapeutic cargo for Parkinson’s disease treatment.
Nat Commun, 9:1305. Co-first authorship.